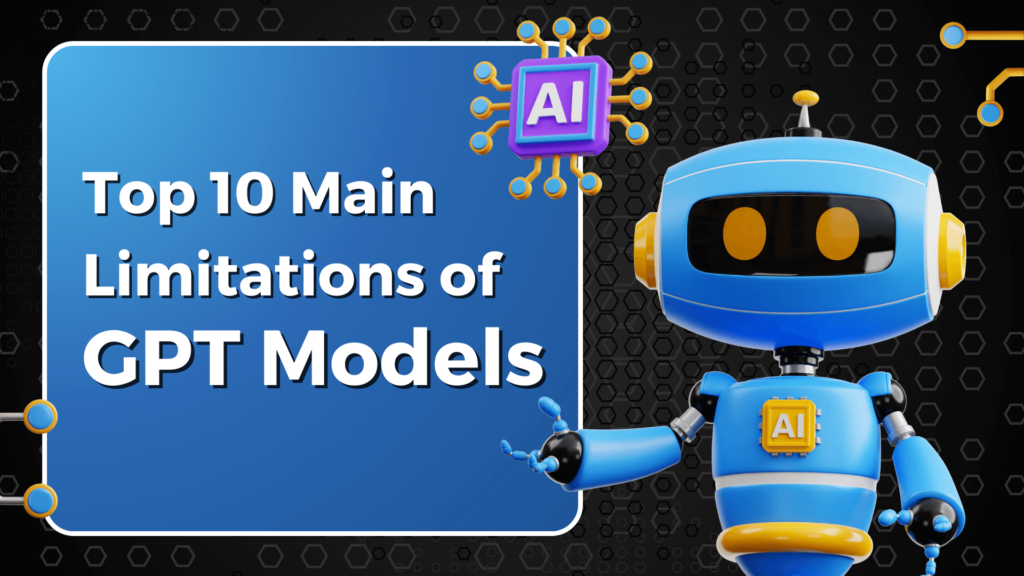
What are the main limitations of GPT models?
The rise of GPT models has significantly changed how we use technology. These high-tech language processors can create text that sounds like someone wrote it, answer questions, and even make up stories. Although they can do amazing things, they also have significant problems that most people need to notice.
GPT models have numerous drawbacks, including a lack of understanding, biases and inconsistencies, factual errors, context awareness, and depending on training data.
Knowing the limitations of GPT models is important for using their power smartly, whether you’re an AI developer, researcher, or just a fan of the technology. Let’s look at some of the main problems with GPT models and discuss what they mean for AI in the future.
Limitation #1: Lack of Common-Sense Knowledge
GPT models are very good at creating text based on data trends. However, they often have trouble with simple, obvious logic. Because of these flaws, GPT models can produce results that don’t make sense or are logically wrong.
When someone asks them something simple like “Can you touch the sky?” Instead of realizing how silly it is to touch something so far away, a GPT model might give a long answer about weather events. This lack of natural understanding can make things more complicated to use in real life. If businesses use these models for customer service, they might need clarification.
Also, users might think that answers are factual advice when, in reality, they’re just repeating patterns they’ve learned without really understanding them. Both developers and users of AI-generated material need to be aware of this gap in reasoning ability.
Limitation #2: Bias in Training Data
GPT models have a significant flaw: the training data can be biased. These systems learn from large data sets showing how people talk and act. This is bad because they can pick up on social biases in the data without meaning.
When skewed data is fed into the model while trained, it changes how the AI responds. For example, racial or gender assumptions might be pushed further instead of being questioned. This happens because the model doesn’t understand; it copies what it has seen.
The consequences are essential. Biased outputs can confuse and keep hurtful stories alive. Users may need to realize where they came from to rely on these correct interpretations.
Both developers and academics need to try to deal with bias. It is important to keep checking datasets for justice and inclusion while also fine-tuning algorithms to have the fewest negative effects on society.
Limitation #3: Difficulty with Out-of-Domain Tasks
GPT models are very good at the things they have been taught to do. However, they may do better when given jobs outside their area of expertise. Patterns from the training data are fundamental for these models to figure out what to do.
The model needs help adapting when the prompt moves into a new area. It might give answers that aren’t useful or make no sense because it needs to understand contexts outside of its training scope. This limitation is evident in areas that need to be precise, like medicine or the law.
Users also usually expect GPT models to work similarly across various topics. This expectation can make people angry when the model fails in places that aren’t well known. As AI improves, closing this gap is still a significant problem for developers and experts.
Limitation #4: Limited Ability to Handle Multimodal Inputs
GPT models are primarily concerned with text-based input. Their architecture must be set up to handle different kinds of info simultaneously. This is a problem for people who want more integrated answers. For example, putting together pictures and words can help people understand many situations. GPT, on the other hand, needs help with jobs that require reading written and visual information.
Its lack of multimodal support makes it less useful in areas where images are important, like education or design. Often, users need different tools for different kinds of information.
As technology improves, there is a greater need for systems that can easily combine different sources. In the future, there may be models that can handle such complexity well. However, for now, this is still a critical limitation of GPT technology.
Limitation #5: Ethical Concerns and Potential Misuse
There are a lot of ethical worries about the rise of GPT models. Their ability to write the text that sounds like someone wrote it can easily be used in evil ways. One big problem is that someone wrote information. This could make campaigns spreading false information stronger or help people write convincing fake news stories that spread false stories.
There is also the chance of making material that supports hate speech or racist ideas. The models could learn and repeat biases found in their training data without meaning, producing immoral results. Another significant issue is privacy. When people use these models, they might give away private information without meaning, making me wonder how the data is kept and used.
Authors and journalists need help with the automation of content creation. It can make people less original and creative while flooding platforms with text generated by computers without natural human intelligence.

Limitation #6: Insufficient Awareness of Context
GPT models are great at creating text but often need to be more consistent regarding context and understand complicated scenarios better. This can lead to people saying things that are entirely off base. For example, a question that can be interpreted in multiple ways might throw these models off. They might come up with an answer that fits one theory but not at all fit another.
Context isn’t just what people say; it’s also what they mean and how they feel. GPT needs help understanding these subtleties, making talks seem disconnected or pointless. Cultural references or local phrases make things even more challenging. When users want funny or valuable information they can relate to, GPT might be unable to deliver.
This lack of knowledge of context makes them less functional in real life, where understanding human feelings and subtleties is essential for good communication.
Limitation #7: Possible Solutions and Future Developments
It is essential to continue studying to fix the problems with GPT models. Developers are looking into better ways to train people to use a more comprehensive range of information. This could cut down on biases a lot and make things more accurate generally.
Putting symbolic reasoning and machine learning together is another exciting idea. Future models can better understand common sense and context if they combine these methods.
When AI makers and ethicists work together, it can lead to more responsible AI use. By setting clear rules, you can reduce the chance of misuse and encourage valuable apps. Also, progress in mixed learning is very encouraging. Models that can handle text, images, or audio can give users a more satisfying interaction experience.
Investing money in user feedback loops will also help models improve over time. By constantly changing based on real-world data, GPT systems can become more reliable tools for many uses.
Limitation #8: The Illusion of Understanding
GPT models can create text that makes sense and fits its surroundings. However, this leads to a common misunderstanding: they do not understand the content they’re creating.
The truth is not like that. These models use patterns they’ve learned from vast amounts of data but need help understanding language or ideas. They might give intelligent answers when asked hard questions, but they often need to catch up on the point.
This false sense of understanding can be a problem in essential situations. Users might put too much faith in information produced by AI without realizing it’s just intelligent copying and not real insight.
As long as we keep using these technologies, it’s important to remember that polished words are made by a program responding to commands, not a mind that can reason or think critically. This shows how important it is to have human control when AI outputs are interpreted.
Limitation #9: Data Dependency and Bias
The data keeps GPT models alive. How well they work depends on how good and varied the training data is. If this data shows biases, those biases can affect the model’s results. For instance, a dataset that mostly includes people from one group could produce skewed representations in the content. This raises moral questions about what is fair and includes everyone.
Also, if certain points of view are emphasized heavily in the training, other points of view might not be covered at all. For this reason, the results are often not balanced.
People who use these models to get information or develop new ideas need to be aware of any biases that might be built into them. Being aware of these limitations helps you think critically when using material made by AI.
Limitation #10: Sensitivity to Prompting and Input
GPT models are very responsive to commands and information. Words can make a huge difference in how things turn out. This variety shows how complicated language is and how these models understand it.
For example, if you ask a question one way, you might get a thorough answer, but if you rephrase it, you might get something short or unrelated. This sensitivity makes it clear that GPTs don’t naturally understand the context.
This reliance on specific language makes it hard for users to engage since they might need to understand how important the way they type is. You must be very careful when writing questions to get the desired results.
This trait also raises concerns about uniformity. If the model answers similar questions differently, its reliability needs to be clarified. Users often look for straightforward answers but have to deal with uncertainty instead.
Limitation #11: Limited Creativity and Originality
GPT models are very good at making up text, but their imagination is often limited. These systems get a lot of their ideas from data that already exists. In other words, they can keep making different versions of what has already been shown.
The limits become apparent when you have to develop something genuinely original. The model can remix ideas but needs help developing groundbreaking new ideas or stories. It lacks the human touch that leads to natural creativity.
Also, these models don’t have any personal feelings or memories they can use. They don’t use their instincts or have a unique point of view, so the results are formulaic and sometimes need more inspiration.
This makes me wonder about their role in creative fields like art and writing. They can help you develop ideas, but depending on them alone might not get you far. It would help if you had a spark that AI can’t replicate to be genuinely unique.
Limitation #12: Dependency on Computational Resources
It takes a lot of computing power to run GPT models. For these large-scale systems to be trained, they need modern hardware and a lot of resources. This often makes it harder for people to get to, especially for smaller groups or individual workers.
Another worry is the high amount of energy used. Running these models can use a lot of electricity and hurt the environment. This point must be remembered as the world pays more attention to ecology.
Real-time systems may also have problems with latency. When GPT models need to respond quickly, it can strain the infrastructure already in place, making them less useful in situations where speed is important.
Scalability is another problem that needs to be solved. As the number of users increases, keeping speed high gets harder and uses more resources. Finding the right balance between efficiency and capability is still a big problem for developers dealing with these technologies.
Limitation #13: The Future of GPT Models: Overcoming Limitations
The future of GPT models looks bright, but some problems must be fixed. Researchers are eagerly looking for ways to improve these models’ ability to help people understand. By adding knowledge graphs and making it easier to remember what was said, we could improve their ability to understand and write text that sounds like someone wrote it.
It is essential to deal with data sharing. Diversifying training datasets are being worked on to reduce the problematic biases in earlier versions. This will make the outputs fairer and generate content representing a broader range of points of view.
Commonsense thinking is still an area open to new ideas. Combining statistical learning and logical frameworks could help us understand more about complicated situations or subtleties in language. Adaptive algorithms that learn to better understand human intent and context over time can also make prompts more sensitive, making them easier to use and understand.
Conclusion: Limitations of GPT Models
Natural language processing is changing quickly, and GPT models are at the forefront of the pack. However, it’s essential to know the limitations of GPT models to be used effectively and help them grow in the future.
For example, not knowing enough about common sense or having biased training data affects performance and dependability in their own ways. The model finds it hard to handle jobs outside its domain and needs help to handle multimodal inputs, limiting its usefulness.
Concerns about ethics are also crucial since the wrong use of artificial intelligence could negatively affect society. Factual errors and the need to understand the context better can make people distrust AI-generated content.
To solve these problems, we need to keep researching and developing new ideas. Improvements are on the way, so there’s hope that future versions will fix the issues with the current ones and open up even more options for GPT technology.